Ai analytics in security cameras
There have been several recent advancements in AI analytics for security cameras that aim to improve surveillance and threat detection capabilities. Here are a few examples:
Object Detection and Recognition: AI algorithms can now accurately detect and recognize objects in camera footage, such as people, vehicles, and even specific types of objects like guns. This can help security personnel quickly identify potential threats and respond accordingly.
Behavior Analytics: AI can analyze patterns of behavior in camera footage and identify anomalies that may indicate suspicious or criminal activity. For example, if someone is loitering in a certain area for an extended period of time, the AI may flag it as a potential security threat.
Facial Recognition: Facial recognition technology is becoming increasingly sophisticated, allowing cameras to accurately identify individuals in real-time. This can be useful for identifying suspects or potential threats in crowded areas.
Predictive Analytics: By analyzing historical camera footage and other data sources, AI can predict potential security threats before they occur. This can help security personnel take proactive measures to prevent security incidents.
Natural Language Processing: AI algorithms can now analyze audio from security cameras and other sources, using natural language processing to identify specific keywords or phrases that may indicate a potential security threat.
Overall, these advancements in AI analytics for security cameras are helping to improve the efficiency and effectiveness of security operations, making it easier to identify and respond to potential threats in real-time.
how does Object detection and recognition works on security camera?
Object detection and recognition on security cameras typically involves using computer vision algorithms to analyze camera footage and identify objects within the scene. The process usually involves the following steps.
Image Preprocessing: The camera footage is first preprocessed to ensure that the images are of good quality and to remove any noise or distortion that may affect object recognition.
Object Localization: The algorithm then locates the objects within the image by drawing bounding boxes around them. This is done using various techniques such as sliding window detection, region-based detection, or anchor-based detection.
Object Classification: The algorithm then analyzes the objects within the bounding boxes and classifies them into specific categories, such as people, vehicles, or animals. This is done using machine learning models trained on large datasets of labeled images.
Object Tracking: Once the objects have been detected and classified, the algorithm may also track their movements within the scene over time.
Object detection and recognition can be used for a variety of applications in security, such as identifying intruders or detecting suspicious objects. It can also be used to trigger alarms or alerts when certain objects are detected, allowing security personnel to respond quickly to potential threats.
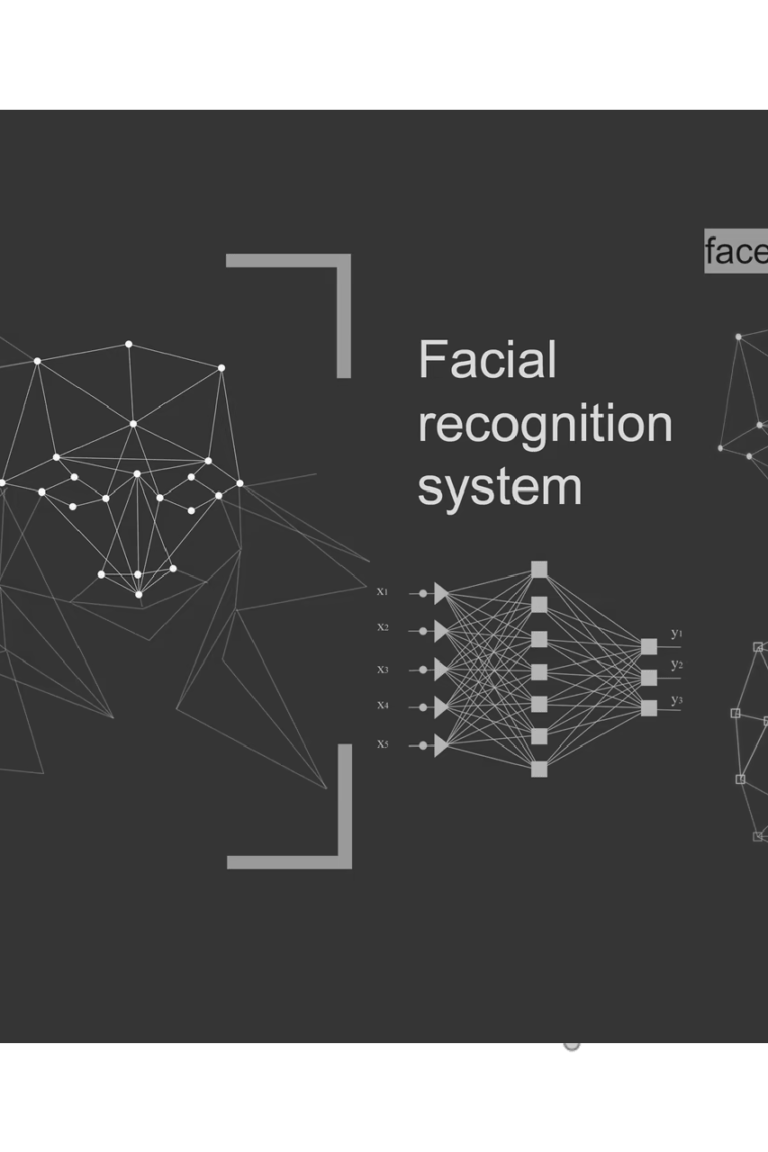
Facial recognition algorithms used in security cameras typically work in the following steps:
Detection: The algorithm scans the video feed from the security camera and detects any faces present in the footage. This is done by analyzing the various features of the face, such as the distance between the eyes, the shape of the nose and mouth, and the overall structure of the face.
Alignment: Once a face has been detected, the algorithm attempts to align it so that it is facing forward and centered within the frame. This step is important because it ensures that the facial features are all in the same position, which makes it easier for the algorithm to compare them to a reference image.
Feature Extraction: The algorithm then extracts various features from the aligned face, such as the distance between the eyes, the shape of the eyebrows, and the curvature of the lips. These features are used to create a unique numerical representation of the face, which is called a facial template.
Comparison: The facial template generated by the algorithm is then compared to a database of known faces to see if there is a match. The database can include images of individuals who have been previously identified or have been added to the system for monitoring. The algorithm calculates a similarity score between the facial template and the images in the database to determine if there is a match.
Decision: Based on the similarity score, the algorithm makes a decision as to whether the face in the video feed matches any of the faces in the database. If there is a match, the system can be programmed to take various actions, such as alerting security personnel or unlocking a secure area.
It is worth noting that facial recognition algorithms are not foolproof and can sometimes produce false positives or false negatives. Therefore, they should be used as a tool to aid security personnel rather than as a replacement for human judgement.
How is behavior analysis executed on security camera?
Behavior analysis on security cameras typically involves using software to analyze video footage in real-time or after the fact, to detect unusual or suspicious behavior patterns. The software uses algorithms to identify behaviors that deviate from normal or expected patterns, such as loitering, unusual movements, or people moving in restricted areas.
The process typically involves setting up the security cameras in strategic locations to capture as much of the area as possible, and configuring the software to analyze the video feeds. The software may be trained to detect specific behaviors based on a set of pre-defined rules, or it may use machine learning algorithms to learn from past footage and identify new patterns.
Once the behavior analysis software identifies an abnormal behavior, it can alert security personnel in real-time or store the footage for review later. This can help security personnel take proactive measures to prevent criminal activity or investigate incidents after they occur.
Overall, behavior analysis on security cameras can be a powerful tool for enhancing security, as it can help identify potential security threats before they turn into more serious incidents. However, it is important to note that the effectiveness of the system depends on the quality of the video footage and the accuracy of the behavior analysis algorithms.